AI vs Generative AI: Unraveling the Future of Technology

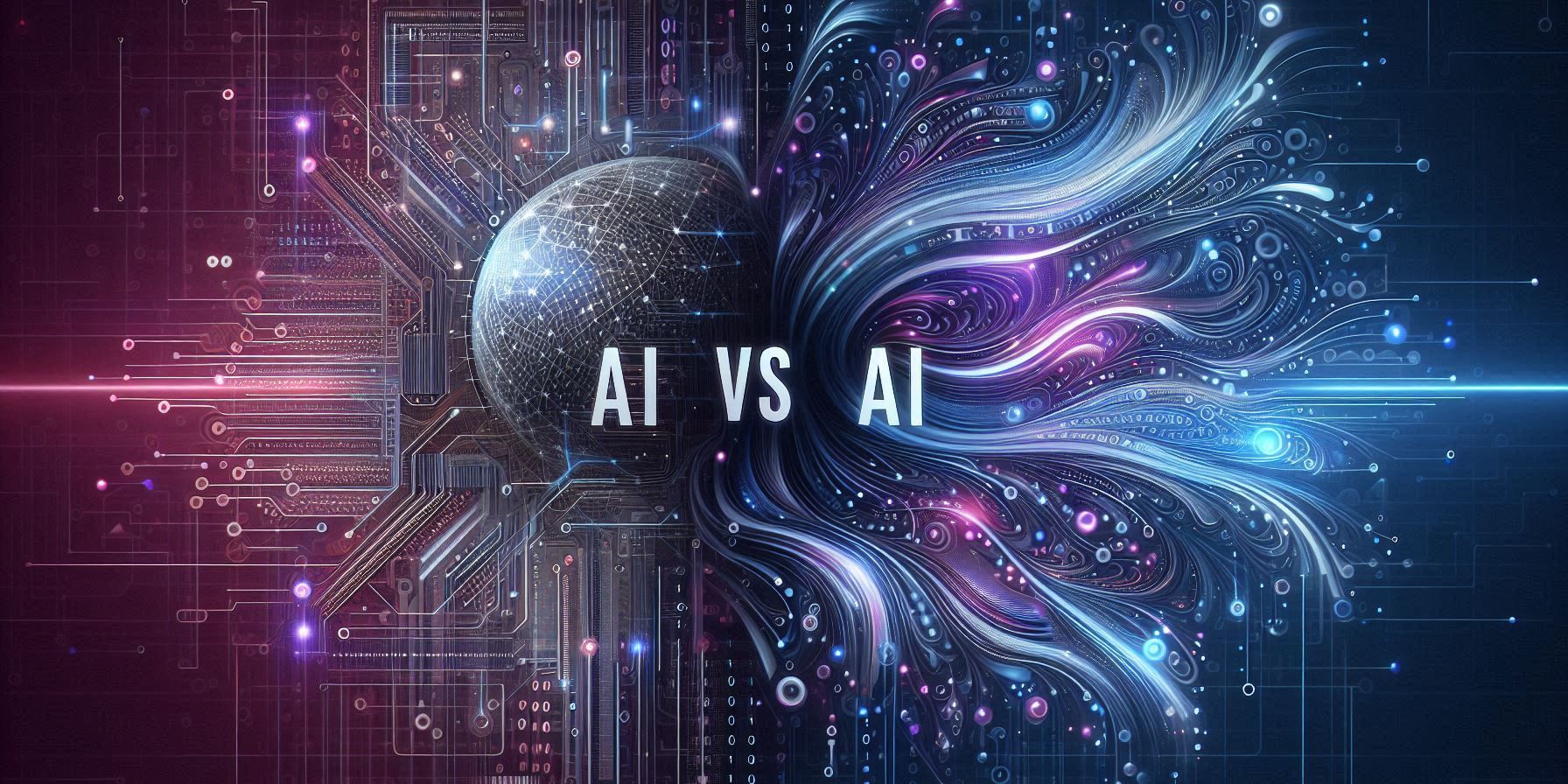

AI vs Generative AI: Unraveling the Future of Technology
Artificial Intelligence (AI) has been shaping the technology landscape for decades. However, with the rise of generative AI, the conversation has become more dynamic, creating new possibilities and raising questions about the future. In this blog post, we’ll take a beginner-friendly journey to understand what AI and generative AI are, how they differ, and their role in technology today.
What is Artificial Intelligence?
Artificial Intelligence, commonly referred to as AI is a branch of computer science that focuses on creating machines capable of performing tasks that typically require human intelligence. These tasks range from problem-solving and decision-making to understanding language and recognizing patterns. AI systems are designed to simulate cognitive functions, allowing computers to analyze data, make predictions, and even learn from experience.
There are two main types of AI:
- Narrow AI: Also known as Weak AI, it is designed to perform a specific task, such as facial recognition or language translation. It cannot operate beyond its programmed function.
- General AI: This represents the idea of machines that can mimic human intelligence across a wide range of tasks. While still largely theoretical, General AI aims to replicate human thought processes entirely.
The Evolution of AI
The concept of AI has been around since the mid-20th century. Over time, the field has grown, fueled by advancements in computing power, data availability, and algorithms. Here's a brief look at the key phases in AI development:
- Early Days: In the 1950s, AI began as a theoretical field, with researchers like Alan Turing introducing foundational ideas about machine learning and intelligence.
- Expert Systems: During the 1980s, AI moved toward rule-based systems, where computers used predefined knowledge to solve problems in specific domains, such as medical diagnostics.
- Machine Learning: In the 2000s, AI experienced significant growth with the rise of machine learning, where computers could analyze large datasets and identify patterns without needing explicit programming.
- Deep Learning and Neural Networks: By the 2010s, deep learning, a subset of machine learning, emerged. Neural networks that mimic the human brain allow machines to learn complex tasks like image recognition and natural language processing.
Large Language Models (LLMs)
A breakthrough in AI has been the development of Large Language Models (LLMs). These models, such as OpenAI's GPT (Generative Pretrained Transformer), are designed to understand and generate human-like text based on vast amounts of data.
LLMs are trained on millions or even billions of pieces of text, allowing them to generate coherent, contextually accurate, and sometimes even creative responses to a wide variety of prompts. These models form the backbone of many advanced AI systems today and are used in chatbots, content generation, translation, and more.
The true power of LLMs lies in their ability to understand the nuances of language, context, and meaning, making them incredibly versatile in both business and everyday applications.
Natural Language Processing (NLP)
NLP, or Natural Language Processing, is a crucial component of AI that focuses on enabling machines to understand, interpret, and respond to human language. From voice assistants like Siri and Alexa to translation tools and customer service bots, NLP allows computers to engage with humans in more natural and intuitive ways.
Some of the key tasks that NLP enables are:
- Text analysis: Extracting information and understanding the sentiment or intent behind a piece of text.
- Speech recognition: Converting spoken words into text, as seen in voice assistants.
- Language translation: Automatically converting text or speech from one language to another.
- Text generation: Creating new content based on a prompt, which is central to generative AI.
What is Generative AI?
Generative AI is a specialized branch of AI focused on creating new content—whether it’s text, images, music, or even videos—by learning patterns from existing data. Unlike traditional AI, which might be used to classify or analyze data, generative AI actively creates.
For example, tools like DALL-E and GPT (both from OpenAI) can generate realistic images or long-form text based on simple prompts. This has vast implications across industries, including art, design, writing, entertainment, and more.
While generative AI is still rooted in the broader concept of AI, its ability to produce original outputs based on learned patterns distinguishes it as a powerful tool in today’s tech landscape.
Examples and Use Cases
Now, let's dive into some practical examples and use cases of both traditional AI and generative AI:
1. Healthcare
- Traditional AI: AI is widely used in diagnostics and predictive analytics, helping doctors identify diseases from medical images (e.g., X-rays or MRIs) or predict patient outcomes based on historical data.
- Generative AI: AI-generated molecules are being tested in drug discovery, creating new compounds that could lead to innovative treatments for various diseases.
2. Entertainment
- Traditional AI: AI systems help recommend content on platforms like Netflix or Spotify by analyzing user preferences and consumption patterns.
- Generative AI: AI tools like OpenAI's ChatGPT or DALL-E are used to write scripts, generate images, or even compose music, reducing creative bottlenecks.
3. Customer Service
- Traditional AI: AI chatbots are commonly used to handle customer queries, providing automated responses based on pre-programmed logic.
- Generative AI: Advanced chatbots powered by generative AI can engage in more complex, human-like conversations, learning from user interactions to improve responses over time.
4. Marketing and Content Creation
- Traditional AI: AI is used for data analysis and targeted advertising, helping businesses optimize campaigns based on customer behavior.
- Generative AI: Tools like Jasper AI or Writesonic can generate marketing copy, blog posts, and even social media content, allowing marketers to create more content faster than ever before.
Conclusion
AI and generative AI represent two exciting branches of technology that are reshaping the future. While AI has long been integral in fields like healthcare, finance, and customer service, generative AI is unlocking new possibilities in creative industries, education, and beyond.
As AI continues to evolve, its applications will only grow more advanced, with the line between machine and human creativity increasingly blurring. Understanding these technologies is essential as they continue to transform industries and shape our daily lives.